Unit 3: AI Ethics & Governance
Comprehensive Overview of Ethical Considerations & Governance Frameworks For AI
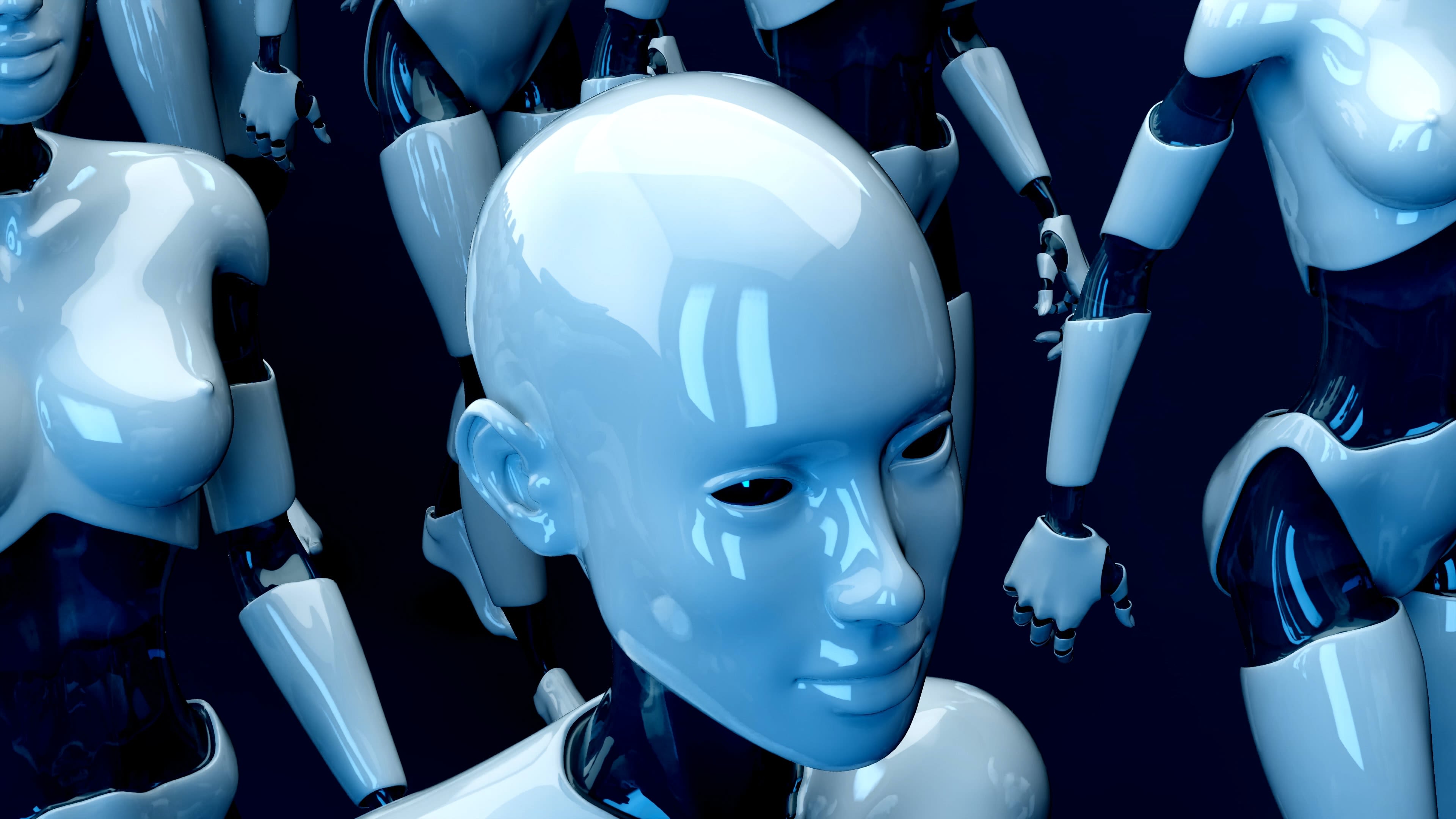
As Artificial Intelligence continues to expand in the world, what is going to be considered safe, fair and just?
AI Ethics and Governance
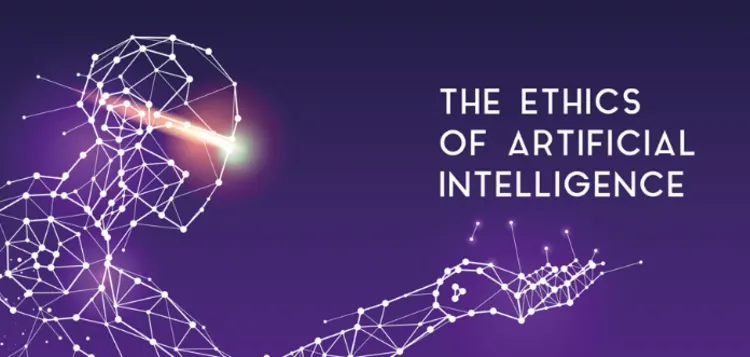
Ethics
Ethical AI is crucial to prevent discrimination, bias, and harm to individuals or groups that may result from AI systems. Engineers must proactively assess datasets and algorithms to identify potential biases and ensure transparency, while companies should have oversight processes to monitor AI's societal impacts. Promoting diversity among teams developing AI can also help reduce biased outcomes, along with enacting regulations that hold organizations accountable for unethical AI that violates civil liberties or infringes on human rights.
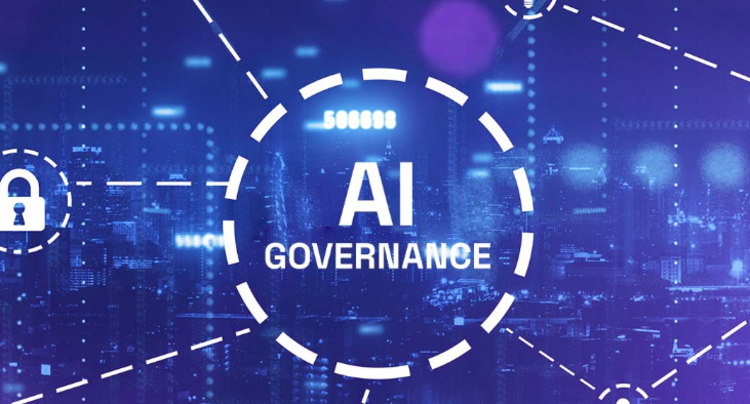
Governance
AI governance refers to the set of principles, guidelines, and practices that ensure the responsible and ethical development, deployment, and use of artificial intelligence technologies. This involves assessing AI systems for potential risks of biases, discrimination, loss of privacy, and other harm to individuals or society. To uphold ethical AI, organizations need transparency, accountability processes, diverse development teams, and partnerships between stakeholders in the public, private, and non-profit sectors to create enforceable regulations and standards.
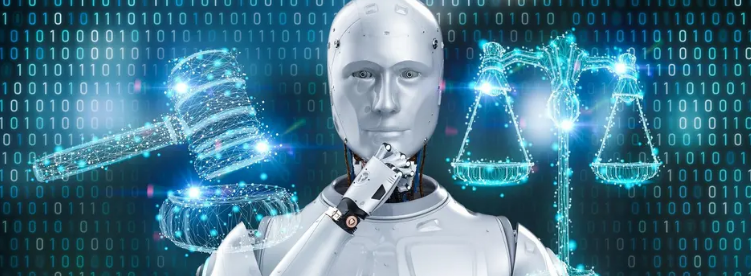
AI Ethics
Fairness, bias, and transparency are crucial components of AI ethics
Fairness
Fairness generally means the absence of prejudice or favoritism towards individuals or groups based on their characteristics.
Fairness in math and in AI has three deferent versions, all important to keeping a model as fair as possible:
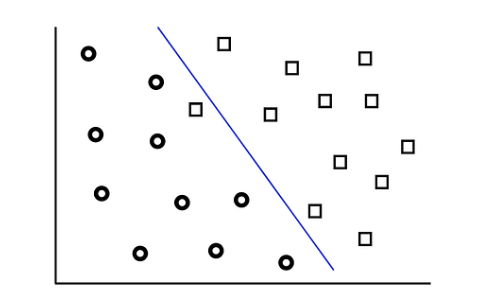
Let's say this graph with circles and squares has a blue line that is considered the most fair for both sides.
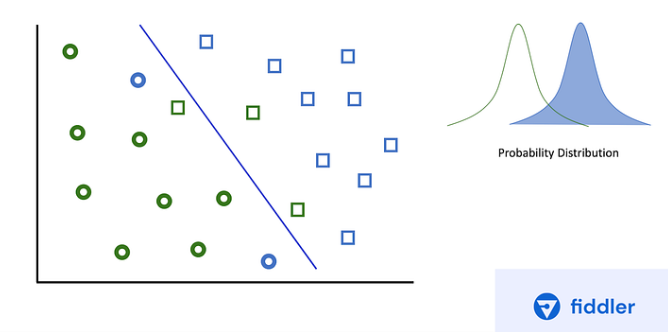
Now this graph has green for people that have diabetes, and blue for people that do not. As you can see only a few people overlap for both probability distributions. Using a single threshold for these two groups would lead to poor health outcomes.
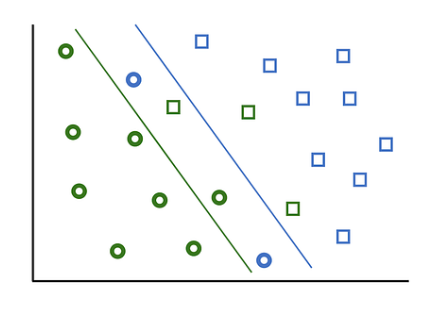
A common thought of what to do is to use calibrated predictions for each group. Yet in this graph you can see that if your model’s scores aren’t calibrated for each group, it’s likely that you’re systemically overestimating or underestimating the probability of the outcome for one of your groups. With various groups doing this is impossible.
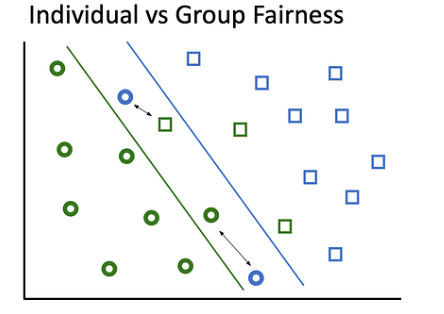
This problem leads to other issues called individual fairness. the black arrows point to blue and green individuals that share very similar characteristics, but are being treated completely differently by the AI system. As you can see, there is often a tension between group and individual fairness.
Ensuring fairness is a critical ethical priority in AI development and deployment. Algorithmic bias can emerge from issues in data collection, such as non-representative sampling, as well as during model training if systems learn to replicate and amplify existing prejudices. This could result in outputs that discriminate against certain demographics in harmful ways, for instance in loan application approvals, employee hiring, criminal sentencing, and more. Promoting fairness requires testing systems for disparate impact on vulnerable groups, diversifying data and teams developing AI, and being transparent about limitations and uncertainty in predictions. Ongoing oversight is necessary and regulations must evolve to protect individuals and communities against unethical AI systems that reinforce injustice. Organizations have a responsibility to proactively assess AI for fairness, implement bias mitigation strategies, and increase access to AI technology and skills across all parts of society.
Bias
Bias is leaning in favor or against something, typically in a way that is closed-minded, prejudicial, or unfair. Bias results in inequitable views and treatment of people or things, where one is given advantage over others.
Also, AI bias refers to discrimination, prejudice, or unfairness that arises in AI systems' outputs and predictions due to ingrained human biases in the data or assumptions used to train machine learning models. When flawed human decisions, historical inequities, stereotypes, or imbalanced variables around factors like race or gender exist in training data or algorithms, they imprint those same biases into the models.
World
Bias perpetuates inequality and injustice in society. Implicit biases are automatic associations that influence our split-second decisions. These unconscious stereotypes privilege some groups over others, affecting real world outcomes. Overt bias also endures through discriminatory policies, rhetoric, and hate crimes that marginalize people based on race, gender, age, and other characteristics. While some progress has been made, addressing both implicit and explicit bias remains critical for promoting compassion and equality.
Data
For AI to learn they need data to be fed. These datasets are both structured and unstructured datasets. However the main cause of bias is from the datasets that they are getting fed. Training data can bake in human biases, while collection and sampling choices may fail to represent certain groups. User-generated data can also create feedback loops that amplify bias. Algorithms identify statistical correlations in data that can be unacceptable or illegal to use, like race or gender.
AI/ML
Even if we have perfect data, our modeling methods can introduce bias.
Aggregation bias: Inappropriately combining distinct groups into one model. Fails to account for differences between groups. Example - Using one diabetes prediction model across ethnicity when HbA1c levels differ.
An example of this is: A machine learning algorithm may also pick up on statistical correlations that are socially unacceptable or illegal. (mortgage lending model)
Human Review
Even if your model is making correct predictions, a human reviewer can introduce their own biases when they decide whether to accept or disregard a model’s prediction. For example, a human reviewer might override a correct model prediction based on their own systemic bias, saying something to the effect of, “I know that demographic, and they never perform well.”
However, AI can reduce humans’ subjective interpretation of data, because machine learning algorithms learn to consider only the variables that improve their predictive accuracy, based on the training data used. In addition, some evidence shows that algorithms can improve decision making, causing it to become fairer in the process.
Actions
Although bias can "never" be solved due to unsolvable capitalism, social, political, and cultural viewpoints, there are various strategies to lower the amount of bias in artificial intelligence.
One example that can contribute is that the internet is mostly created by the majority rather than the minority. Our history books were created by the majority "altering" the history. The majority will never want anyone to see themselves as the problem. This is continued in the production of AI since the majority are leading this new field.
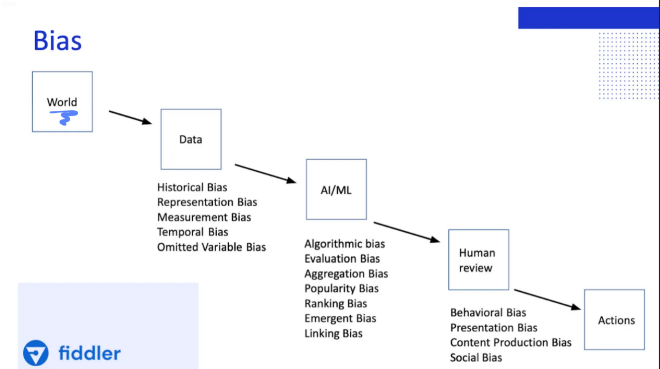
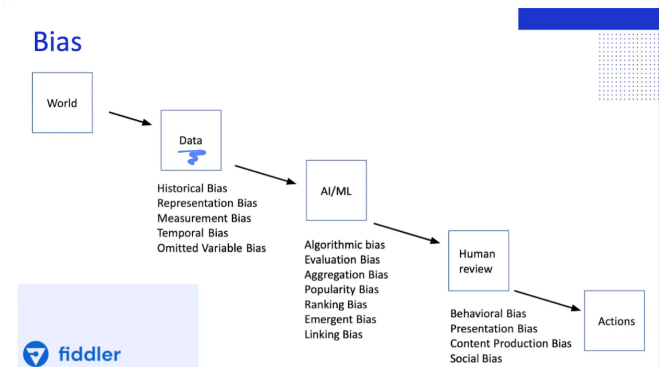
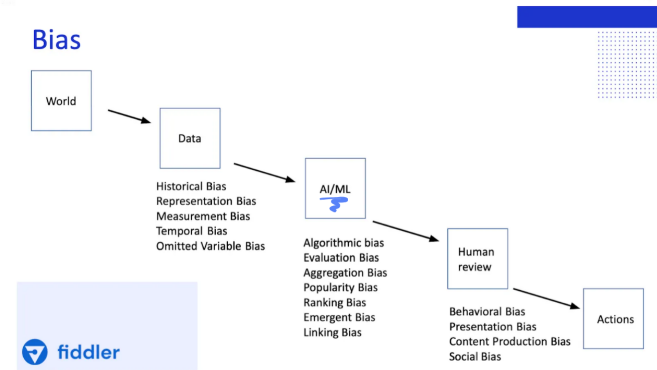
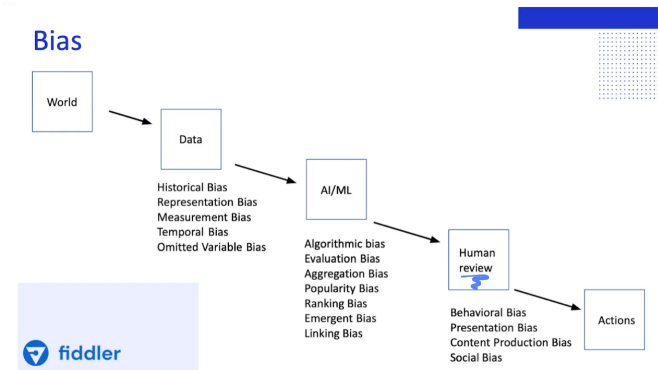
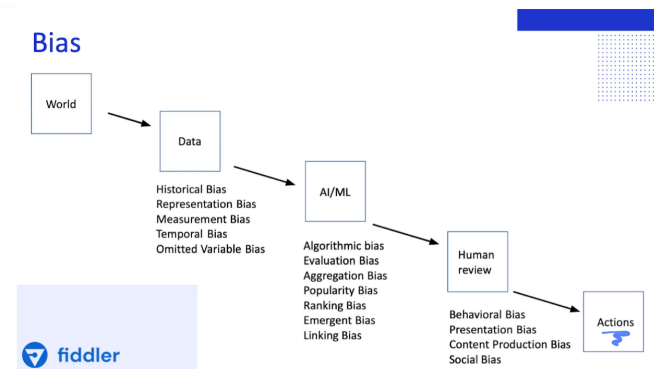
Transparency
Transparency is the quality of being open, honest, and easily understood, allowing for clear visibility and comprehension of actions, decisions, or processes.
Transparency is a key element of ethical AI because it builds trust and accountability. When the data sources, processing methods, algorithmic models, and limitations of AI systems are openly communicated and inspectable, it enables monitoring for issues like bias. Opaque “black box” systems that lack explainability present risks, as negative impacts on individuals or society may go unseen. Being transparent about AI capabilities and shortcomings, and providing clear explanations for outputs and recommendations, is important so human operators can make informed decisions. Users should know when they are interacting with an AI system versus a human and be empowered to challenge inappropriate or biased results. Fostering transparency will involve creating standards around documentation, audits, and accessibility. Ethical AI demands transparency both to improve system development and to uphold the human rights and interests of those affected by AI.
AI Privacy and Invasion
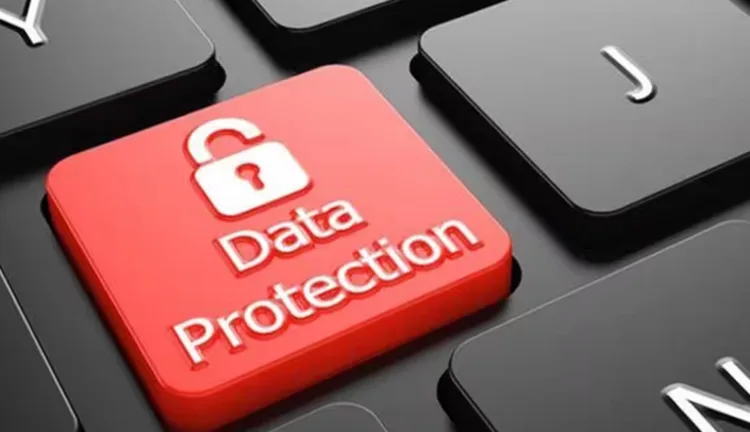
Data Protection and Privacy Concerns
The use of AI technologies often involves the collection, analysis, and processing of vast amounts of personal data, raising concerns about the potential invasion of privacy. Safeguarding against unauthorized access, data breaches, and ensuring compliance with privacy regulations become critical in maintaining individual rights and protecting sensitive information.
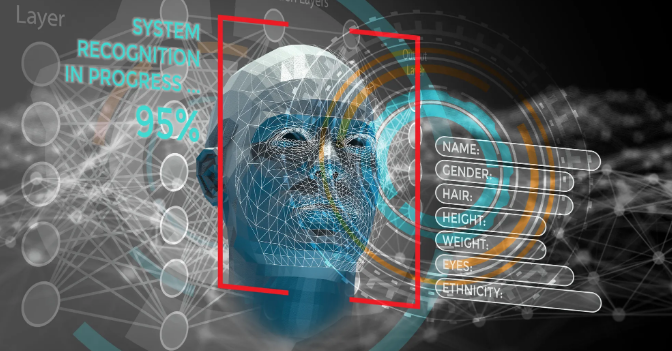
Surveillance and Ethical Considerations
AI applications in surveillance, such as facial recognition and monitoring systems, raise ethical questions regarding the balance between security and privacy. The deployment of AI for surveillance purposes can lead to the invasion of personal spaces and threaten civil liberties, necessitating careful consideration of the ethical implications and the establishment of responsible usage guidelines.
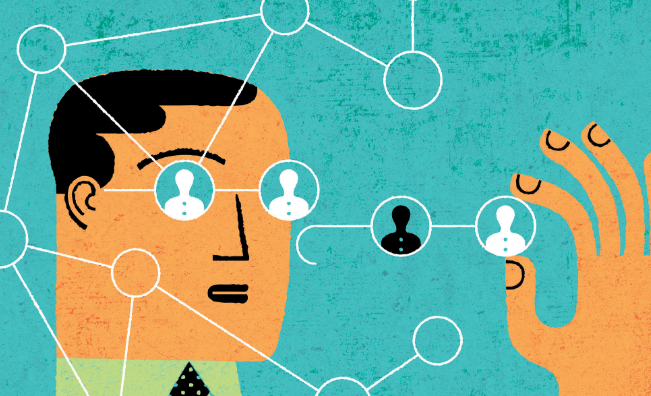
Algorithmic Bias and Discrimination
The development and deployment of AI algorithms may inadvertently perpetuate biases present in training data, leading to discriminatory outcomes. This bias can result in an invasion of privacy, particularly for marginalized groups, as automated systems may make decisions based on sensitive attributes. Addressing algorithmic bias and ensuring fairness in AI systems are essential to mitigate privacy concerns and uphold ethical standards.
Regulatory Landscape
Government Regulations:
Industry Standards:
Ethical Frameworks:
Conclusion
The ethical considerations surrounding AI, encompassing issues of bias, fairness, transparency, and privacy, underscore the imperative for responsible development and deployment of artificial intelligence systems. Recognizing and mitigating algorithmic biases is essential to ensure fair and just outcomes, promoting inclusivity and preventing discrimination. Transparency in AI processes fosters accountability, enabling stakeholders to comprehend and scrutinize the decision-making mechanisms. Privacy concerns demand robust safeguards against unauthorized access and data breaches, safeguarding individual rights in an increasingly interconnected world. By adhering to ethical principles, the integration of AI can be a force for positive transformation, empowering societies to harness the benefits of technology while upholding fundamental values and respecting the dignity of individuals. As the AI landscape evolves, a commitment to ethics remains paramount in navigating the intricate balance between technological advancement and the preservation of human values.
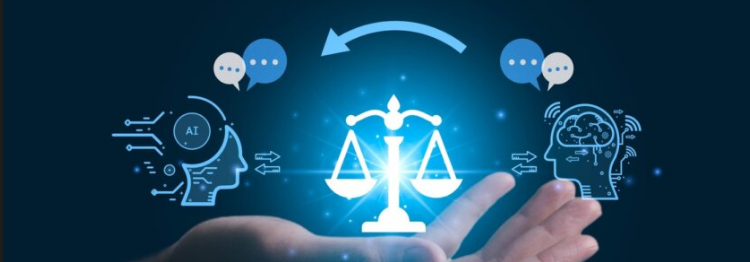
AI Governance
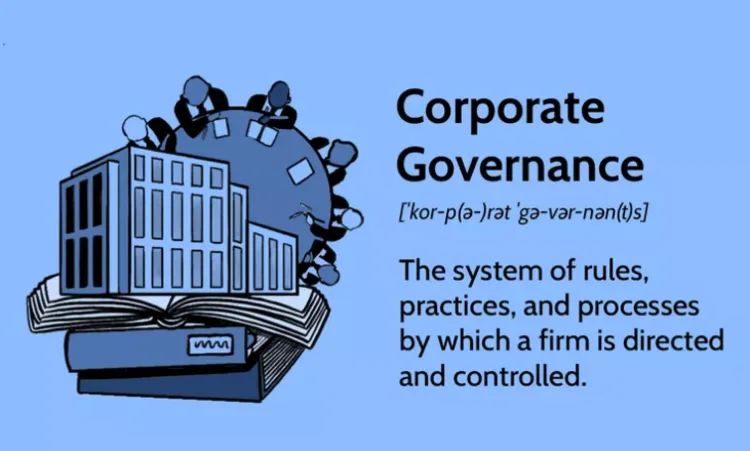
Corporate Governance
Responsible AI requires dedicated oversight within organizations to enact ethical practices. Companies should create review boards with cross-functional leaders to evaluate AI systems before deployment for potential risks or harms. Technical teams should consult checklists based on core principles during development to minimize possible biases in data or algorithms. Conducting impact assessments and audits enables monitoring of real-world performance and continuous improvement. HR must integrate ethics training into professional development, and legal/compliance teams should guide policy creation that upholds transparency and accountability. Diversity is critical in governance roles to represent diverse viewpoints on societal impacts. Though internal structures alone cannot guarantee ethical AI, they provide necessary infrastructure to assess tradeoffs, implement guidelines, and operationalize principles. With comprehensive governance and stakeholder participation, companies can build trust in AI and prevent unacceptable outcomes that violate human rights.
Benefits:
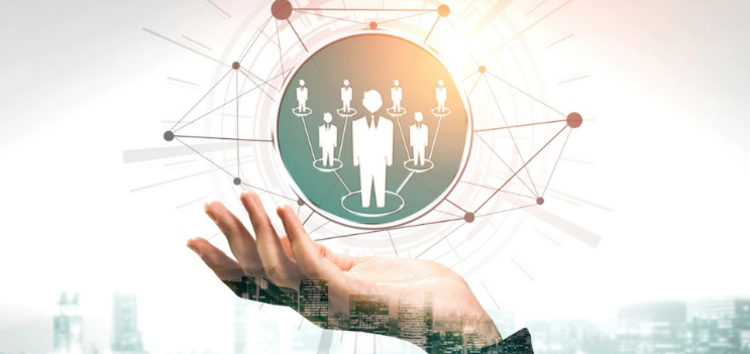
Multistakeholder Collaboration
A multi-stakeholder approach is necessary to effectively govern AI in the public interest. Governments can consult experts in drafting regulations that protect society while supporting innovation. Companies must commit to self-regulation and compliance beyond what laws require. Academics can research AI impacts and recommend evidence-based policies. Civil society groups, media, and the public provide crucial oversight and advocacy around AI ethics. Industry associations should encourage members to adopt voluntary standards. Global cooperation will aid consistency across borders. With diverse inputs, compromise can be found balancing different viewpoints and priorities. Partnerships between stakeholders enable leveraging complementary strengths for research, funding, guidelines, policymaking and enforcement. By sharing accountability, reasonable standards emerge and pressure mounts for transparent, unbiased and socially-conscious AI. Combined top-down and bottom-up efforts from various sectors of society reinforce ethical norms and create an ecosystem governing AI for the common good.
Benefits
Strategies:
The Anglo-American Model - corporate governance model, exemplified by the Shareholder Model, gives primary control to shareholders and the board of directors while providing incentives to align management goals. Shareholders elect board members and vote on key decisions, encouraging accountability through potential withdrawal of financial support. Regulators in the U.S. tend to favor shareholders over boards and executives. Ongoing communication facilitates oversight and shareholder participation.
The Continental Model - has a two-tier structure with separate supervisory and management boards. The supervisory board contains outside shareholders, banks, and other stakeholders who oversee the insider executives on the management board. This model aligns with government objectives and highly values stakeholder engagement.
The Japanese Model - concentrates power with banks, affiliated companies, major shareholders called Keiretsu, insiders, and the government. It offers less transparency and focuses on the interests of those key players rather than minority shareholders.
Example:
Volkswagen AG
Volkswagen AG faced a severe crisis in September 2015 when the "Dieselgate" scandal exposed the company's deliberate and systematic manipulation of engine emission equipment to deceive pollution tests in the U.S. and Europe. This revelation led to a nearly 50% drop in the company's stock value and a 4.5% decline in global sales in the subsequent month. The governance structure played a significant role in facilitating the emissions rigging, as Volkswagen's two-tier board system lacked the independence and authority necessary for effective oversight. The supervisory board, intended to monitor management and approve corporate decisions, was compromised by a large number of shareholders, with 90% of voting rights controlled by board members, undermining its ability to act independently. This lack of effective supervision allowed the illegal activities to persist, highlighting the critical importance of robust corporate governance and ethical oversight in preventing such scandals.
Challenges
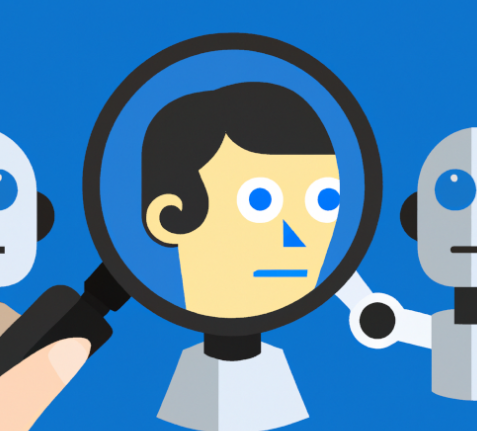
Lack of Standards
The field of AI currently lacks a single, globally-adopted set of ethical guidelines, presenting obstacles to consistent practices. With various standards emerging from different public and private sector organizations, inconsistencies and gaps arise on issues like transparency, bias mitigation, and accountability. Companies may abide by certain principles but ignore others without unified norms. Enforcement also proves difficult without formal regulations requiring compliance. This allows questionable AI uses to slip through the cracks. The borderless nature of AI systems means differing rules across countries enable exploitation of loopholes. Competing priorities of advancing innovation versus minimizing harm leads to delays in aligning standards. Until consensus builds around core ethical values encoded in mandatory universal standards, uncertainty and conflicts will persist, enabling unethical AI deployment despite preventable harms. Global leadership and collaboration is necessary to develop a unified ethical AI framework and overcome discrepancies that now allow irresponsible practices.
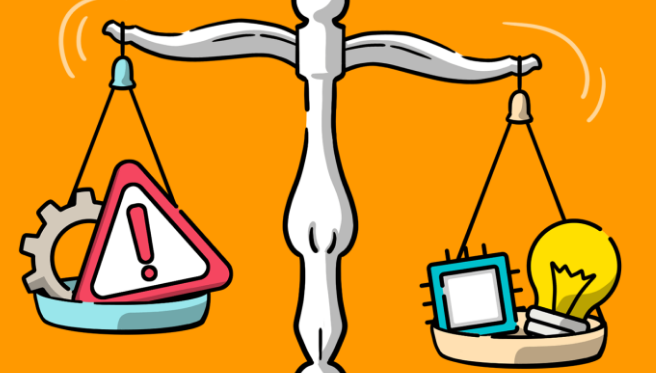
Balancing Innovation and Responsibility
Balancing AI innovation and ethical responsibility poses complex tradeoffs. Unrestrained innovation risks unacceptable harms, while onerous ethics regulations may stifle progress of beneficial technologies. Companies want rapid advancement and competitive advantage, but governments and civil groups prioritize public welfare. Formulating policies that both shepherd advances and proactively mitigate risks requires nuance and compromise between these interests. There are no blanket solutions, as the appropriate equilibrium depends on context-specific factors like the technology's maturity level, potential applications and abuses, and cultural frameworks. Continuous cost-benefit analyses of principles like transparency, accountability and bias mitigation is required to find optimal flexibility for innovators without compromising human rights. Multi-stakeholder collaboration that accounts for diverse viewpoints can identify reasonable governance guardrails. Though challenging, balanced policies that further progress responsibly are imperative for AI serving humanity's shared values.
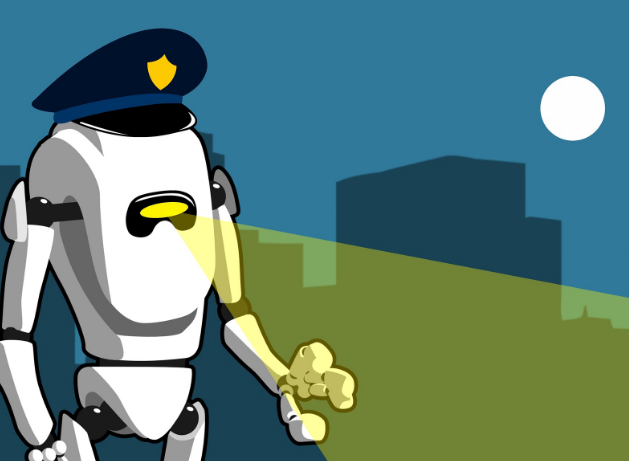
Enforcement Issues
Enforcing adherence to AI ethics and regulations is difficult. Voluntary principles lack accountability, while laws can lag behind technological change. Opacity of complex AI systems obscures violations. Regulatory resources to oversee vast tech sectors may be limited. Conflicting rules across jurisdictions enable loophole exploitation. Companies prioritizing profits over ethics resist external oversight or pursue minimum compliance. Lack of incentives, audits and penalties allow willful ignorance of harms. Technical literacy is required for nuanced enforcement. Rapid evolution of AI systems outpaces governance updates. Compliance is only possible through clear regulations, incentives for responsible development, transparency requirements, proactive audits, adept oversight bodies, and collaborations with ethical AI leaders pursuing accountable practices.
ChatGPT doing it right!
"my worst fear is that we cause significant, we the field the technology of the industry cause significant harm to the world if this technology goes wrong it can go quite wrong and we want to be vocal about that we want to work with the government to prevent that from happening but we try to be very clear eyed about what the downside case is and the work that we have to do to mitigate that. " private tech ceo asking for more government regulation "I'm nervous about it I think people are able to adapt quite quickly when photoshop came out to the scene a long time ago you know for a while people were really quite fooled by photoshop images and pretty quickly developed an understanding that images might be photosphopped uh this will be like that but on steroids." - Sam Altman
AI Future Trends
As AI expands into different fields, the imperative for robust ethical considerations and governance frameworks becomes evident, emphasizing transparency, fairness, and accountability to ensure responsible development and deployment of AI technologies across various industries.
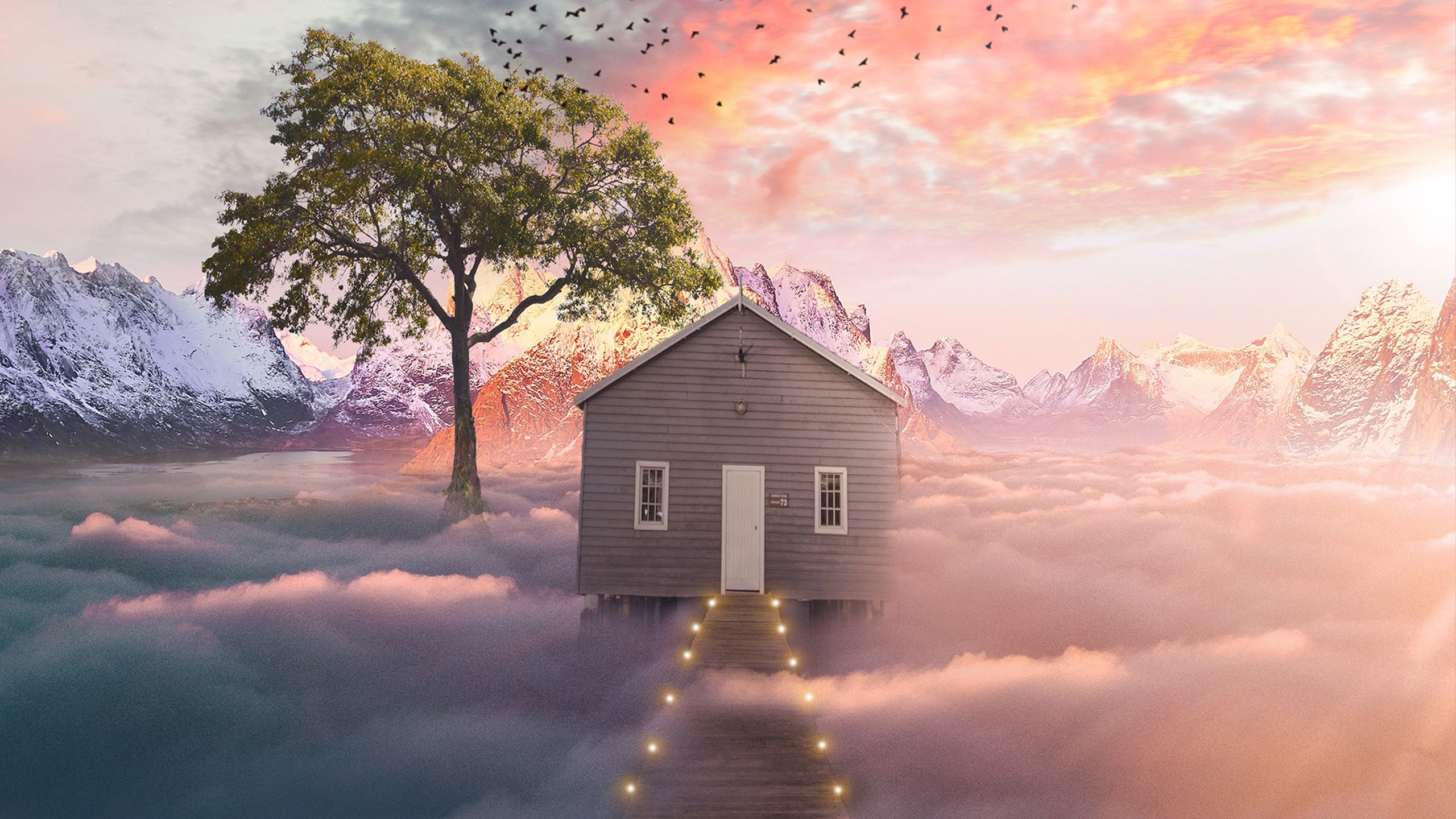
Education
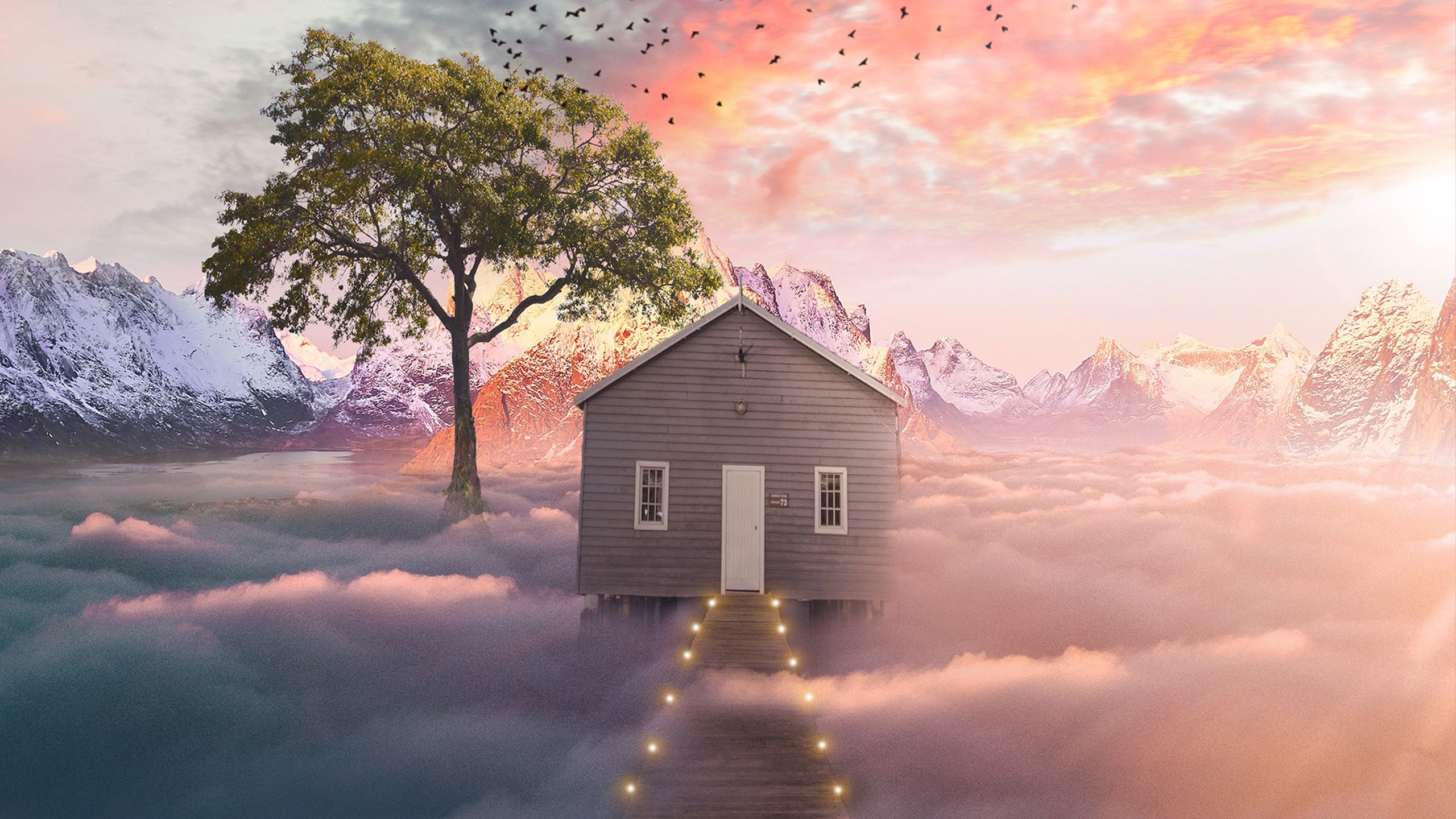
Workforce
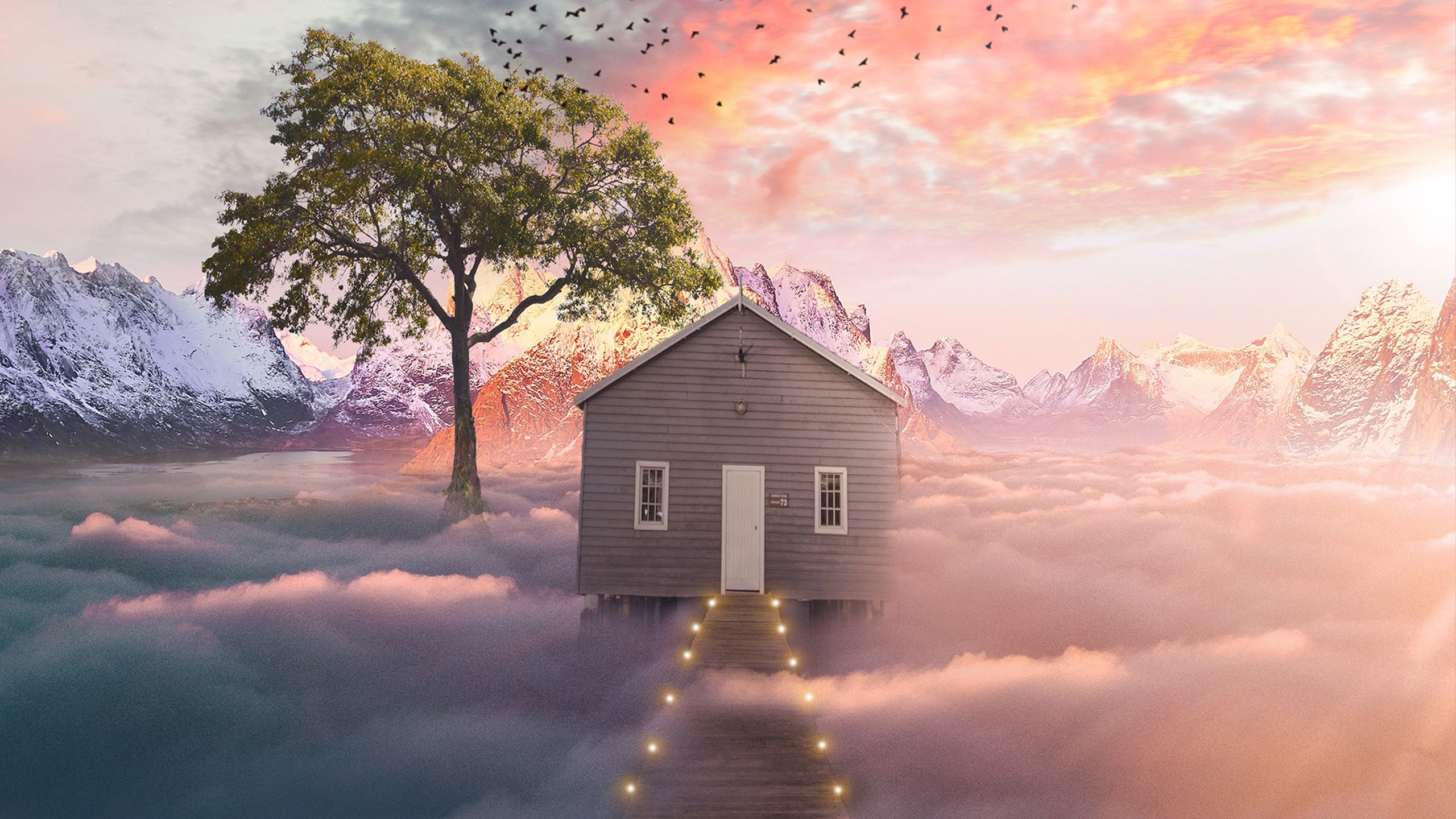